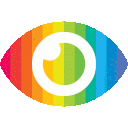
1. Hong Kong has severe air quality problems due to high population and traffic densities.
2. Principal component analysis (PCA) and cluster analysis (CA) can be used to optimize the air quality monitoring network.
3. PCA was used to identify areas with similar air pollution behavior for SO2, RSP, and NO2, while CA was applied to group stations based on similarity for RSP and NO2 pollutants.
The article titled "Performance assessment of air quality monitoring networks using principal component analysis and cluster analysis" discusses the use of PCA and CA to optimize the air quality monitoring network in Hong Kong. The article highlights the severe air quality problems in Hong Kong due to its high population and traffic densities. The article also mentions that HKEPD has established fourteen monitoring stations equipped with various instruments for detecting corresponding pollutants.
The article provides a detailed explanation of PCA and CA, their applications, and how they can complement each other to provide a practical alternative approach for analyzing and solving environmental problems. The article cites several previous studies that have used these two approaches to explore significant information from the origin data.
The article then goes on to describe the materials and methods used in this study, including sample location, available data, PCA, and CA. The results of the study are presented in Table 2, which shows the main results of PCA analysis at all stations for pollutants of SO2, RSP, and NO2.
While the article provides valuable insights into how PCA and CA can be used to optimize air quality monitoring networks, it has some potential biases. For example, the article only focuses on SO2, RSP, and NO2 pollutants while ignoring other pollutants that may also contribute to air pollution. Additionally, the article does not mention any potential risks associated with using PCA and CA or any limitations of these approaches.
Furthermore, while the article cites several previous studies that have used PCA and CA successfully, it does not explore any counterarguments or limitations of these approaches. This one-sided reporting may lead readers to believe that these approaches are infallible when they may have their own set of limitations.
In conclusion, while this article provides valuable insights into how PCA and CA can be used to optimize air quality monitoring networks in Hong Kong, it has some potential biases such as one-sided reporting and ignoring other pollutants that may contribute to air pollution. Therefore readers should approach the article with a critical eye and consider other sources of information before drawing any conclusions.