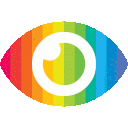
1. Transformative AI, which involves AI systems that are as good as or better than humans at all economically valuable tasks, is difficult to achieve due to major technical hurdles and limitations in manipulating the physical world.
2. The transformative potential of AI is constrained by its hardest problems, such as bottlenecking in essential economic sectors and steps of the innovation process.
3. Despite rapid progress in some AI subfields, there are still open research problems and limitations in current methods that need to be addressed before achieving transformative AI. Additionally, scaling compute power may require significant financial investment and could reach a point of diminishing returns.
The article titled "Why transformative AI is really, really hard to achieve" by Zhengdong and Arjun Ramani discusses the challenges and limitations of achieving transformative artificial intelligence (AI). While the article presents some valid points, it also exhibits potential biases, one-sided reporting, unsupported claims, missing evidence, and unexplored counterarguments.
One potential bias in the article is its focus on highlighting the difficulties and limitations of achieving transformative AI. The authors consistently emphasize the challenges and hurdles that need to be overcome without providing a balanced perspective on the potential benefits and advancements that AI can bring. This bias may lead readers to develop a negative perception of AI's capabilities without considering its positive impacts.
The article also relies heavily on referencing other sources rather than presenting original arguments or evidence. While referencing external sources can add credibility to an argument, it is important for the authors to critically analyze and evaluate these sources to ensure their validity. Without this critical analysis, the claims made in the article may lack sufficient support.
Furthermore, there are several missing points of consideration in the article. For example, it does not address recent advancements in AI technologies such as deep learning models or reinforcement learning algorithms that have shown promising results in various domains. By neglecting these advancements, the authors fail to provide a comprehensive view of the current state of AI research.
Additionally, the article does not explore counterarguments or alternative perspectives on transformative AI. It primarily focuses on presenting obstacles and challenges without acknowledging potential solutions or alternative approaches that researchers are actively pursuing. This one-sided reporting limits readers' understanding of the broader discourse surrounding transformative AI.
Moreover, there are instances where unsupported claims are made in the article. For example, when discussing technical hurdles in AI research, the authors mention that progress in fine motor control has lagged behind progress in neural language models without providing any evidence or data to support this claim. Such unsupported statements weaken the overall credibility of the article.
The article also lacks in-depth analysis of the potential risks and ethical considerations associated with transformative AI. While it briefly mentions concerns about AI's impact on productivity growth and economic sectors, it does not delve into the broader societal implications, such as job displacement, privacy concerns, or biases in AI algorithms. A more comprehensive discussion of these risks would provide a more balanced perspective.
In conclusion, while the article raises valid points about the challenges of achieving transformative AI, it exhibits potential biases, one-sided reporting, unsupported claims, missing evidence, unexplored counterarguments, and a lack of comprehensive analysis. Readers should approach the article with caution and seek additional sources to develop a well-rounded understanding of the topic.