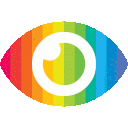
1. Multivariate time series forecasting is a fundamental problem attracting many researchers in various fields, but most existing methods focus on univariate prediction and rarely take into account the potential spatial dependencies between multiple variables.
2. A novel graph neural network model based on multi-scale temporal feature extraction and attention mechanism is proposed for multivariate time series prediction, which can take full advantage of the interdependence between time series while preserving temporal relationships.
3. Empirical mode decomposition is used to extract the time-domain features of multivariate time series at different time scales to form the node features of the graph, and the multi-head attention mechanism is applied to construct potential associations between nodes and enhance the rationality of relationships in the graph.
作为一篇科技论文,该文章的内容相对客观,但仍存在一些潜在的偏见和局限性。
首先,文章提到了传统的多元时间序列预测方法存在线性假设的问题,并且随后介绍了基于深度学习的方法。然而,文章并没有探讨这些深度学习方法是否也存在某些假设或局限性。此外,文章没有提及其他可能的预测方法,如基于统计模型或机器学习算法的非深度学习方法。
其次,文章强调了图神经网络在处理关系依赖性方面的有效性,并将其应用于多元时间序列预测中。然而,在实际应用中,图神经网络也存在一些限制和挑战。例如,在处理大规模图数据时,计算复杂度可能会很高;在缺少足够标记数据时,如何进行有效训练也是一个问题。
此外,在介绍所提出模型时,文章没有详细说明每个组件之间的关系和作用方式。例如,在使用多头注意力机制时,如何选择注意力头数目以及如何确定权重分配等问题都需要进一步解释和探讨。
最后,在实验部分中,虽然作者使用了真实数据进行验证,并取得了良好结果。但是作者并没有探究该模型在不同场景下的适用性和鲁棒性,并且未考虑到可能存在的风险因素。
总之,尽管该论文提出了一种新颖且有前景的多元时间序列预测方法,并取得了良好结果。但是,在阅读和引用该论文时需要注意其潜在偏见和局限性,并结合其他相关研究进行评估和比较。