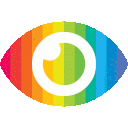
1. Machine learning (ML) has been adopted in vehicular networks for applications such as autonomous driving, road safety prediction and vehicular object detection.
2. Federated learning (FL) is an efficient tool that reduces transmission overhead while achieving privacy through the transmission of model updates instead of the whole dataset.
3. This paper investigates the usage of FL over CL in vehicular network applications to develop intelligent transportation systems.
The article provides a comprehensive analysis on the feasibility of federated learning (FL) for machine learning based vehicular applications, as well as investigating object detection by utilizing image-based datasets as a case study. The article does not present any counterarguments or alternative points of view, which could be beneficial in providing a more balanced perspective on the topic. Additionally, there is no evidence provided to support some of the claims made in the article, such as “Federated learning (FL) is an efficient tool that reduces transmission overhead while achieving privacy through the transmission of model updates instead of the whole dataset”. Furthermore, there is no discussion on potential risks associated with using FL in vehicular networks, such as security risks due to data leakage or malicious attacks on edge devices. The article also does not explore other possible solutions for reducing transmission overhead and improving privacy in ML based vehicular applications. In conclusion, this article provides a detailed overview of federated learning in vehicular networks but fails to provide sufficient evidence and explore alternative solutions or potential risks associated with its use.