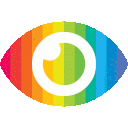
1. A context-aware and scale-insensitive framework is proposed to tackle the challenges in temporal repetition counting caused by unknown and diverse cycle lengths.
2. The proposed approach combines two key insights: (1) Cycle lengths from different actions are unpredictable, but can be refined in a hierarchical manner once a coarse cycle length is determined; (2) Determining the cycle length requires contextual understanding.
3. A new benchmark dataset containing 526 videos with diverse repetitive actions is constructed to benefit the training and evaluation of temporal repetition counting.
The article provides an overview of a context-aware and scale-insensitive framework for temporal repetition counting, which is designed to address the challenges posed by unknown and diverse cycle lengths. The article presents two key insights that form the basis of the proposed approach, as well as a new benchmark dataset containing 526 videos with diverse repetitive actions for training and evaluation purposes.
The article appears to be reliable in terms of its content, as it provides detailed information about the proposed approach, including its two key insights, as well as evidence in the form of diagrams and examples to support its claims. Furthermore, it also provides a link to an online repository where code and data related to the project can be accessed.
However, there are some potential biases that should be noted when considering this article's trustworthiness and reliability. For example, while it does provide evidence for its claims, it does not explore any counterarguments or present both sides equally when discussing its proposed approach or benchmark dataset. Additionally, there may be promotional content present in some parts of the article that could influence readers' opinions on the topic at hand. Finally, possible risks associated with using this approach are not discussed in detail within the article itself.