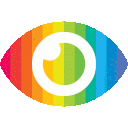
1. Federated Learning is a machine learning technique that distributes model training over mobile user equipments (UEs), allowing each UE to independently compute the gradient based on its local training data. This technique ensures data privacy and can involve a large number of participants with powerful processors and low-delay mobile-edge networks.
2. The article highlights the need to address challenges in Federated Learning, such as uncertainty of wireless channels, heterogeneous power constraints of UEs, and varying local data sizes. These challenges impact trade-offs between computation and communication latencies, as well as the time required for Federated Learning and UE energy consumption.
3. To tackle these challenges, the article proposes an optimization problem called FEDL that captures the trade-offs mentioned above. Despite being non-convex, the problem is decomposed into three convex sub-problems, for which closed-form solutions are obtained. These solutions provide insights into problem design by determining optimal learning time, accuracy level, and UE energy cost. Extensive numerical results are also provided to examine various factors affecting Federated Learning over wireless networks.
Overall, the article emphasizes the benefits and challenges of Federated Learning in wireless networks and presents an optimization model to address these challenges effectively.
这篇文章介绍了一种名为联邦学习的机器学习技术,该技术利用多个移动用户参与模型训练。文章指出,联邦学习可以保护用户数据隐私,并且具有大量用户参与和低延迟的优势。然而,文章也提到了一些问题,如无线信道的不确定性、用户设备功耗和本地数据大小的异质性等,这些问题对计算和通信延迟之间的权衡产生影响。
文章提出了一个名为FEDL的优化问题模型来解决这些问题,并通过将其分解为三个凸子问题来获得全局最优解。作者还通过数值结果进行了理论分析。
然而,这篇文章存在一些潜在的偏见和片面报道。首先,文章没有充分探讨联邦学习可能面临的隐私风险。虽然文章提到了保护用户数据隐私是联邦学习的一个优点,但没有深入讨论可能存在的数据泄露或滥用风险。
其次,文章没有平等地呈现双方观点。它主要关注联邦学习的好处和应用前景,但没有提及任何潜在的缺点或限制。
此外,在提出FEDL模型时,文章没有提供足够的证据来支持其主张。虽然作者声称通过分解问题并求解子问题可以获得全局最优解,但没有提供实际的数值结果或实验证明这一点。
最后,文章没有探讨可能存在的风险和挑战。例如,联邦学习涉及多个参与者共享模型更新,这可能导致恶意攻击或篡改数据的风险。文章也没有讨论如何处理不可靠的无线信道或用户设备之间的异质性。
综上所述,这篇文章在介绍联邦学习技术和提出FEDL模型方面做出了一定的贡献,但存在一些潜在的偏见和不足之处。进一步研究和实证分析是必要的,以更全面地评估联邦学习技术的优势、限制和潜在风险。