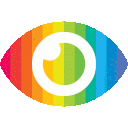
1. Thermal comfort in buildings affects occupants’ productivity and quality of life, as well as building energy consumption.
2. The Predicted Mean Vote (PMV) model is widely used to predict thermal comfort, but it is not effective in naturally ventilated buildings.
3. Machine learning algorithms, such as artificial neural networks and support vector machines, can be used to predict occupants’ thermal comfort in naturally ventilated residential buildings using field survey data.
The article "Using machine learning algorithms to predict occupants’ thermal comfort in naturally ventilated residential buildings" provides an overview of the current methods used to predict thermal comfort in buildings and proposes the use of machine learning algorithms to improve accuracy. While the article presents a comprehensive review of previous research, it has some potential biases and limitations.
One potential bias is that the study only focuses on residential buildings in China, which may limit its generalizability to other regions with different climates and building types. Additionally, the study only considers natural ventilation conditions, which may not be applicable to all buildings or situations.
The article also presents some unsupported claims, such as stating that machine learning algorithms have "excellent self-learning abilities" without providing evidence or examples. The article could benefit from more detailed explanations of how machine learning algorithms work and their potential limitations.
Furthermore, while the article compares different models for predicting thermal comfort, it does not provide a thorough analysis of their strengths and weaknesses. For example, while the PMV model is widely used, it has been criticized for not accurately predicting thermal comfort in naturally ventilated buildings. However, the article does not explore why this is the case or how other models address this limitation.
The article also lacks discussion of potential risks associated with using machine learning algorithms for predicting thermal comfort. For example, relying solely on algorithmic predictions may overlook important factors that affect occupants' comfort and well-being.
Overall, while the article provides a useful overview of current research on predicting thermal comfort in buildings and proposes a promising approach using machine learning algorithms, it could benefit from more critical analysis and discussion of potential limitations and biases.