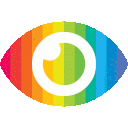
1. Ready-IoT is a novel forensic readiness model for IoT environments that collects provenance from the network to aid in constructing artifacts during forensic investigations.
2. The model generates Link-IoT dataset by querying provenance graphs, which can be used to detect attacks performed on the IoT network.
3. The proposed model primarily uses provenance information alongside network traffic to derive features listed in Link-IoT dataset, which distinguishes it from existing datasets used for research in traditional computer networks.
The article "Ready-IoT: A Novel Forensic Readiness Model for Internet of Things" proposes a proactive forensic readiness model for IoT networks based on network provenance. The article highlights the need for forensic solutions in IoT environments due to the increased risks to security and privacy. The proposed model collects and analyzes the behavior of nodes to identify suspicious events and generates Link-IoT dataset, which can aid in detecting attacks performed on the IoT network.
The article provides a comprehensive review of existing forensic models designed for IoT environments, highlighting their strengths and weaknesses. However, it is important to note that the article primarily focuses on proactive approaches rather than reactive ones. This bias may limit the effectiveness of the proposed model in real-world scenarios where reactive approaches are often necessary.
The article also discusses various datasets developed to evaluate intrusion detection solutions designed for IoT networks. However, it fails to provide a detailed analysis of these datasets' limitations and how they may impact the proposed model's effectiveness.
Furthermore, while the article acknowledges that Ready-IoT is confined to the proactive phase defined in the holistic model proposed in [11], it does not provide any insights into how this limitation may impact its effectiveness in real-world scenarios.
Overall, while the proposed model has potential benefits, it is important to consider its limitations and biases when evaluating its effectiveness in real-world scenarios.