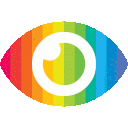
1. The Internet of Things (IoT) is a platform that provides pervasive access and distributed sharing to visualize data in digital format through wireless sensors, machine-to-machine communication, human-computer interfaces, etc.
2. Blockchain-based security for authentication and authorization has been adopted in the IoT to deliver distributed trust, reduce computational complexity in security, and provide swift transactions.
3. Machine learning paradigms assist in identifying adversaries and attacks in an IoT platform by performing intense data analysis from fetching and validating systems logs, blockchain information assessment, etc.
The article titled "Internet of things forensic data analysis using machine learning to identify roots of data scavenging" discusses the use of blockchain technology and machine learning in identifying adversaries and attacks in IoT platforms. The article provides a comprehensive overview of the challenges faced by IoT infrastructure units and service providers in providing seamless service due to security and privacy-related factors.
The article highlights the use of blockchain-based security for authentication and authorization in the IoT, which can deliver distributed trust, reduce computational complexity in security, and provide swift transactions. However, the article fails to mention any potential risks associated with blockchain technology, such as scalability issues or energy consumption concerns.
The proposed framework focuses on administering security for virtualized smart city resources by identifying the roots of data scavenging attacks imposed on virtual machines. The performance evaluation of the proposed framework is conducted using the Contiki Kooja simulator, which simulates 120 IoT devices accessing 48 VRs. However, there is no discussion on how this simulation environment accurately represents real-world scenarios.
The article also mentions that machine learning paradigms assist eventual and less-complex assessment and examination of precise information through differential methods. However, there is no discussion on how these methods may be biased or inaccurate due to incomplete or biased training data.
Overall, while the article provides valuable insights into using blockchain technology and machine learning in identifying adversaries and attacks in IoT platforms, it lacks a balanced discussion on potential risks associated with these technologies. Additionally, there are some missing points of consideration regarding the accuracy and bias of machine learning methods used in this context.