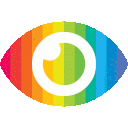
1. Knowledge Graph (KG) is a complex network that contains billions of facts about the real world, represented as triplets of (head entity, relation, tail entity). KG analysis and completion methods are important due to the noise and incompleteness of KGs.
2. Knowledge graph embedding (KGE) aims to embed entities and relations in a low-dimensional vector space for efficient knowledge graph completion. Previous approaches focus on rating each fact in isolation and lack a mechanism to learn the sub-graph structure information of entities in KG.
3. The proposed model, Graph2Seq (G2SKGE), leverages the information fusion mechanism used in graph neural networks to learn fusion embeddings for entities in KG. It specifically aims to learn the sub-graph structure information of entities in KG and outperforms state-of-the-art models in empirical experiments on benchmark datasets.
对于上述文章,我认为它提供了一个关于知识图谱完成的新方法Graph2Seq,并介绍了一个新的KGE模型G2SKGE。然而,文章在以下几个方面存在一些问题:
1. 偏见来源:文章没有提及KG中可能存在的偏见或错误信息。由于KG通常是通过自动化方法构建的,可能会引入错误或不完整的事实。这可能导致模型在预测新事实时出现偏差。
2. 片面报道:文章只关注了使用Graph2Seq和G2SKGE模型来学习实体的子图结构信息,并没有探讨其他可能的方法或技术。这种片面报道可能导致读者对该领域其他相关研究的理解不完整。
3. 缺失考虑点:文章没有讨论KG中潜在的噪声和不完整性对模型性能的影响。由于KG通常是从多个来源收集而来,其中包含了各种各样的错误和缺失信息。这些问题可能会影响模型在预测新事实时的准确性。
4. 缺失证据:文章没有提供足够的实验证据来支持作者所声称的G2SKGE模型优于其他现有模型。虽然作者声称他们在四个基准数据集上取得了有希望的结果,但没有提供详细的实验结果或比较其他模型的性能。
5. 未探索的反驳:文章没有探讨可能存在的反驳观点或对该方法的质疑。这种缺乏对潜在问题和限制的讨论可能导致读者对该方法的实际可行性产生疑虑。
总体而言,尽管文章提出了一个新颖的方法和模型来解决知识图谱完成任务,但它在一些方面存在一些不足之处。进一步的研究和实验证据需要来支持作者所声称的优势,并考虑到KG中可能存在的偏见和不完整性。