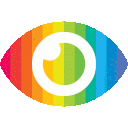
1. An interpolation method is presented for estimating smooth spatiotemporal profiles for local highway traffic variables such as flow, speed, and density.
2. The method is based on stationary detector data and can be augmented by floating car data or other traffic information.
3. The resulting profiles display transitions between free and congested traffic in great detail, as well as fine structures such as stop-and-go waves. The method has potential applications in compensating for gaps in data caused by detector failure, separating noise from dynamic traffic information, and fusing floating car data with stationary detector data.
The article titled "Reconstructing the Traffic State by Fusion of Heterogeneous Data" presents an advanced interpolation method for estimating smooth spatiotemporal profiles for local highway traffic variables such as flow, speed, and density. The authors claim that their method is accurate and robust and can be used to compensate for gaps in data caused by detector failure, separate noise from dynamic traffic information, and fuse floating car data with stationary detector data.
Overall, the article appears to be well-written and informative. However, there are a few potential biases and missing points of consideration that should be addressed.
Firstly, the authors do not provide any information about the limitations of their method. For example, they do not discuss how their method might perform in different weather conditions or how it might be affected by changes in road infrastructure. This lack of information could lead readers to believe that the method is more reliable than it actually is.
Secondly, the authors do not provide any evidence to support their claims about the accuracy and robustness of their method. While they mention that they have established these qualities through testing, they do not provide any details about how this testing was conducted or what specific results were obtained.
Thirdly, the authors appear to be promoting their own work without acknowledging other research in the field. For example, they state that their method is "advanced" without providing any context for what makes it so. Additionally, they do not mention any other methods for estimating traffic variables or compare their own method to existing ones.
Finally, while the authors mention potential applications for their method (compensating for gaps in data caused by detector failure, separating noise from dynamic traffic information, fusing floating car data with stationary detector data), they do not discuss any possible risks associated with using this approach. For example, relying too heavily on interpolated data could lead to inaccurate predictions or decisions being made based on faulty information.
In conclusion, while the article provides valuable insights into a new approach for estimating traffic variables using heterogeneous data sources, there are some potential biases and missing points of consideration that should be addressed before fully accepting its claims.