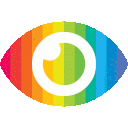
1. Fake news is a growing problem on social media, and it can have negative effects on society by spreading misinformation and manipulating reality.
2. Detecting fake news is a complex process that involves analyzing the content and sentiment of news articles, as well as handling missing data.
3. Multiple imputations are an effective method for handling missing data in fake news research, and machine learning models can be used to detect and classify fake news accurately.
The article titled "Fake news detection in social media based on sentiment analysis using classifier techniques" discusses the problem of fake news and the need for reliable methods to detect it. While the topic is important and relevant, there are several issues with the article that need to be addressed.
Firstly, the article lacks a clear structure and organization. The introduction section jumps from discussing the prevalence of social media to the definition of fake news without providing a smooth transition. This makes it difficult for readers to follow the flow of ideas.
Secondly, the article relies heavily on citations from other sources without providing sufficient analysis or critical evaluation of those sources. Many claims are made without supporting evidence or examples. For example, the article states that fake news always appeals to emotions and discourages rational analysis, but no evidence is provided to support this claim.
Additionally, there is a lack of consideration for potential biases in the research and methods discussed. The article mentions using sentiment analysis as a component of fake news detection, but does not address how biases in sentiment analysis algorithms can affect the accuracy of detection. It also does not explore counterarguments or alternative perspectives on fake news detection.
Furthermore, there is a lack of discussion on potential risks and limitations of using automated systems for detecting fake news. The article assumes that automated systems are necessary due to the large scale of information on social media, but does not address concerns about false positives or censorship.
The article also contains promotional content by mentioning specific studies or models without providing a balanced view of their limitations or criticisms. It presents these studies as solutions without acknowledging potential drawbacks or challenges.
Overall, while the topic of fake news detection is important, this article lacks critical analysis and fails to provide a comprehensive understanding of the subject matter. It would benefit from more thorough research, balanced reporting, and addressing potential biases and limitations in existing methods.