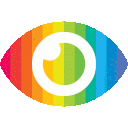
1. MotionDiffuse is a text-driven motion generation framework that uses a diffusion model to generate human motions conditioned on natural languages.
2. The framework offers probabilistic mapping, realistic synthesis, and multi-level manipulation, allowing for diverse and fine-grained motion generation with various text inputs.
3. Experiments show that MotionDiffuse outperforms existing state-of-the-art methods by convincing margins on text-driven motion generation and action-conditioned motion generation, demonstrating its controllability for comprehensive motion generation.
The article titled "MotionDiffuse: Text-Driven Human Motion Generation with Diffusion Model" presents a new framework for generating human motion based on natural language input. The authors claim that their proposed method, MotionDiffuse, outperforms existing state-of-the-art methods in terms of diversity and fine-grained motion generation.
The article provides a clear overview of the problem of human motion modeling and the challenges associated with generating diverse and realistic motions based on text inputs. The authors argue that existing methods are limited by their deterministic language-motion mapping and inability to model complicated data distributions.
However, the article does not provide a comprehensive analysis of the limitations of existing methods or the potential biases in their evaluation. The authors only briefly mention that their experiments show MotionDiffuse outperforms existing methods by convincing margins but do not provide detailed comparisons or statistical analyses.
Furthermore, the article lacks discussion on potential risks associated with using text-driven motion generation frameworks. For example, there may be ethical concerns related to generating realistic motions of individuals without their consent or creating biased representations of certain groups based on the language input used.
Additionally, while the authors claim that MotionDiffuse responds to fine-grained instructions on body parts and arbitrary-length motion synthesis with time-varied text prompts, they do not provide evidence or examples to support these claims. The article also does not explore potential counterarguments or alternative approaches to text-driven motion generation.
Overall, while the article presents an interesting new framework for text-driven human motion generation, it lacks thorough analysis and evidence to support its claims and address potential limitations and risks.