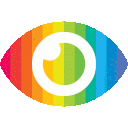
1. Naturalistic reinforcement learning (RL) is a framework that integrates the complexity of real-world environments into the study of human decision-making, providing insights into how humans navigate complex situations.
2. Humans use structure and regularity in naturalistic environments to enable effective and efficient decision-making.
3. Naturalistic priors, context, and task-state representation play crucial roles in supporting human decision-making in real-world environments.
The article titled "Naturalistic Reinforcement Learning: Trends in Cognitive Sciences" discusses the use of naturalistic approaches in studying human decision-making within complex real-world environments. While the article provides valuable insights into the benefits of incorporating naturalism into reinforcement learning (RL) research, there are a few potential biases and limitations to consider.
One potential bias is the focus on RL as the dominant framework for understanding human decision-making. The article presents RL as the central approach within computational cognitive science and computational psychiatry, suggesting that it has been instrumental in recent advances in these fields. However, this perspective overlooks other theoretical frameworks and methodologies that have also contributed to our understanding of decision-making, such as cognitive psychology and behavioral economics. By emphasizing RL as the primary framework, the article may neglect alternative perspectives and potentially limit a comprehensive analysis of human decision-making processes.
Another limitation is the lack of discussion on potential drawbacks or challenges associated with naturalistic RL. While the article highlights the benefits of incorporating complexity from real-world environments into experimental paradigms, it does not adequately address potential risks or limitations. For example, naturalistic environments can introduce confounding variables that may complicate data interpretation and make it challenging to isolate specific cognitive processes. Additionally, replicating real-world complexity in controlled laboratory settings may be difficult and could lead to oversimplification or artificiality. These considerations should be acknowledged to provide a balanced assessment of naturalistic RL.
Furthermore, the article does not thoroughly explore counterarguments or alternative perspectives on naturalistic RL. It primarily focuses on highlighting studies that support its claims about structure and regularity in decision-making within complex environments. However, a more comprehensive analysis would involve discussing studies or theories that challenge or offer different explanations for these findings. By presenting a more balanced view of the existing literature, readers can gain a better understanding of both supporting and opposing evidence regarding naturalistic RL.
Additionally, while the article mentions computational modeling as an important tool in understanding decision-making, it does not provide sufficient evidence or examples of how computational models have contributed to the field. The article could benefit from including specific studies or findings that demonstrate the utility and validity of computational modeling approaches in studying naturalistic decision-making.
Overall, while the article offers valuable insights into the benefits of naturalistic RL, it is important to consider potential biases, limitations, and missing points of consideration. A more balanced analysis that incorporates alternative perspectives, addresses potential risks and challenges, and provides supporting evidence for claims would enhance the overall credibility and comprehensiveness of the article.