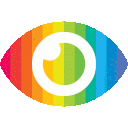
1. Bayesian neural networks provide a rigorous methodology for uncertainty quantification in stock price predictions.
2. The COVID-19 pandemic had a drastic impact on the world economy and stock markets, making it more challenging to provide accurate forecasting.
3. Despite high market volatility during the first peak of the pandemic, Bayesian neural networks were able to provide reasonable predictions with uncertainty quantification.
The article "Bayesian neural networks for stock price forecasting before and during COVID-19 pandemic" presents a study on the use of Bayesian neural networks for stock price prediction, with a focus on uncertainty quantification in predictions. The authors compare their results with state-of-the-art neural network training algorithms and investigate the performance of related forecasting models during the COVID-19 pandemic.
The article provides a clear introduction to the topic and explains the limitations of conventional deep learning methods in terms of uncertainty quantification. The use of Bayesian inference is presented as a solution to this problem, and the authors provide a good overview of MCMC sampling methods for implementing Bayesian neural networks.
However, there are some potential biases in the article that should be noted. Firstly, the authors only consider stocks from four different regions (Germany, China, Australia, and the United States), which may not be representative of global stock markets. Additionally, they only focus on the first phase of the COVID-19 pandemic, which may limit the generalizability of their findings to other periods or future pandemics.
Furthermore, while the authors claim that their results indicate that Bayesian neural networks can provide reasonable predictions with uncertainty quantification despite high market volatility during the first peak of the COVID-19 pandemic, they do not provide enough evidence to support this claim. The article lacks detailed information about how well their model performed compared to other models or benchmarks.
Another potential issue is that while the authors acknowledge that obtaining robust quantification of uncertainty with good prediction accuracy has been a major challenge for effective stock market prediction models, they do not explore counterarguments or alternative approaches to addressing this challenge.
Overall, while this article provides an interesting perspective on using Bayesian neural networks for stock price prediction with uncertainty quantification during COVID-19 pandemic, it would benefit from more comprehensive analysis and evidence to support its claims.