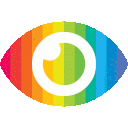
1. Flood forecasting is a critical challenge that requires both accuracy and interpretability in artificial intelligence (AI) models.
2. Existing hydrological solutions lack prediction robustness, while machine learning approaches neglect model interpretability.
3. The proposed Spatio-Temporal Attention Long Short Term Memory (STA-LSTM) model outperforms other models in flood forecasting, and its attention weights provide visualization and interpretation of the model's reasoning.
这篇文章介绍了一个用于洪水预测的可解释的时空注意力LSTM模型。然而,文章存在一些潜在的偏见和问题。
首先,文章声称现有的水文学解决方案在预测稳健性方面表现不佳,而机器学习方法则忽视了模型的物理可解释性。然而,文章没有提供足够的证据来支持这个主张。它没有比较水文学模型和机器学习方法在预测准确性和稳健性方面的实际表现。
其次,文章声称提出的STA-LSTM模型在大多数情况下优于其他模型,如历史平均值、全连接网络、卷积神经网络、图卷积网络等。然而,文章没有提供详细的实验结果或数据来支持这个主张。读者无法评估该模型相对于其他模型的真实效果。
此外,文章没有探讨可能存在的风险或局限性。例如,在使用机器学习方法进行洪水预测时,数据质量和缺失数据可能会对模型的准确性产生重要影响。文章也没有讨论该模型是否适用于不同地区或不同类型的洪水事件。
最后,文章没有平等地呈现双方观点。它只关注了机器学习方法的缺点,而忽视了水文学模型的优点。这种片面报道可能导致读者对洪水预测方法的全面理解。
综上所述,这篇文章在介绍可解释的洪水预测模型方面提供了一些有限的信息,但存在潜在的偏见和不足之处。进一步研究和实证分析是必要的,以评估该模型在实际应用中的效果和可行性。