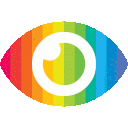
1. Spectral mixture analysis (SMA) is commonly used to derive subpixel vegetation information from remotely sensed imagery in urban areas.
2. Endmember selection is a critical step in SMA, and the spectral signatures of endmembers may vary from pixel to pixel due to changes in biophysical and biochemical composition.
3. The Bayesian Spectral Mixture Analysis (BSMA) model incorporates endmember spectral variability in the unmixing process based on Bayes Theorem, leading to an improved estimate of subpixel vegetation fractions with uncertainty information for the estimates.
作为一篇科学论文,该文章提供了一个关于在城市环境中使用光谱混合分析(SMA)来推导亚像素植被信息的方法。然而,在阅读文章时,我们可以看到一些潜在的偏见和缺失。
首先,文章没有探讨可能存在的风险或负面影响。例如,在城市环境中增加植被可能会导致水资源短缺或者增加昆虫数量等问题。此外,文章也没有平等地呈现双方观点,只强调了植被对城市环境的积极影响。
其次,文章提出了一个新的模型(Bayesian Spectral Mixture Analysis),但并没有提供足够的证据来支持其优越性。作者只是简单地比较了传统SMA和BSMA之间的结果,并得出结论BSMA可以更好地估计亚像素植被分数。然而,这种结论是否适用于其他数据集或场景仍需进一步研究。
此外,在选择端元时,作者只考虑了光谱特征,并未考虑其他因素如空间分布、生物量等。这可能会导致端元选择不准确或不完整。
最后,文章没有探讨如何解决遮挡问题。在城市环境中,建筑物、道路等人造物体可能会遮挡部分植被信息,从而影响SMA结果的准确性。
综上所述,该文章提供了一个有用的方法来推导城市环境中亚像素植被信息。然而,在阅读时需要注意其中存在的偏见和缺失,并需要进一步研究以验证其有效性和适用性。