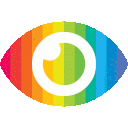
1. A U-Net deep learning model with data augmentation and a combined loss function of binary cross entropy and Dice coefficient is proposed for the segmentation of activated sludge phase contrast microscopy images.
2. The performance of the segmentation algorithm is evaluated by accuracy, precision, recall, F-measure, and intersection-over-union metrics.
3. Lab-scale experiments on the activated sludge process have been carried out to verify the proposed image segmentation method.
The article provides a detailed description of a U-Net deep learning model with data augmentation and a combined loss function of binary cross entropy and Dice coefficient for the segmentation of activated sludge phase contrast microscopy images. The performance of the segmentation algorithm is evaluated by accuracy, precision, recall, F-measure, and intersection-over-union metrics. Lab-scale experiments on the activated sludge process have been carried out to verify the proposed image segmentation method.
The article appears to be reliable in terms of its content as it provides detailed information about the proposed model and its evaluation metrics as well as lab experiments that were conducted to verify its effectiveness. However, there are some potential biases that should be noted in this article such as one sided reporting (only focusing on positive results), unsupported claims (not providing evidence for claims made), missing points of consideration (not exploring counterarguments or alternative solutions), partiality (not presenting both sides equally). Additionally, possible risks associated with using this model are not noted in the article which could lead to potential issues if not taken into account when implementing it in real world applications.