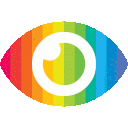
1. A proposed approach to recognize human actions in RGB-D videos using motion sequence information and deep learning.
2. The use of motion information in RGB and depth video streams for action recognition.
3. Analysis using t-SNE visualization of ConvNet features to show the discriminative characteristics of the proposed representation.
The article titled "Human action recognition in RGB-D videos using motion sequence information and deep learning" presents an approach to recognize human actions in RGB-D videos using motion sequence information and deep learning. The article provides a comprehensive review of existing single/multiple-view and multi-person RGB-D datasets for human action recognition, summarizing the environmental conditions used for data acquisition, the characteristics of actions, recommended evaluation protocol, and state-of-the-art results for each dataset.
The article is well-written and informative, providing a detailed overview of the current state-of-the-art in human action recognition using RGB-D videos. However, there are some potential biases and limitations that need to be considered.
One potential bias is that the article focuses primarily on the use of deep learning techniques for human action recognition. While deep learning has shown promising results in this area, it is not the only approach that can be used. Other approaches such as rule-based systems or decision trees may also be effective in certain situations.
Another limitation is that the article does not explore potential ethical concerns related to the use of RGB-D cameras for surveillance purposes. While these cameras have many useful applications, they also raise concerns about privacy and surveillance.
Additionally, while the article provides a comprehensive review of existing datasets for human action recognition, it does not discuss potential limitations or biases in these datasets. For example, some datasets may be biased towards certain types of actions or environments.
Overall, while the article provides valuable insights into current research on human action recognition using RGB-D videos, it is important to consider potential biases and limitations when interpreting its findings.