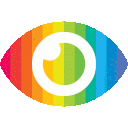
1. Gas turbines (GTs) are essential components of propulsion, power generation, and mechanical-drive systems in the aviation and energy industries.
2. Data-driven modeling methods, such as statistical methods and computational intelligence, are used to analyze GT systems and improve their reliability and profitability.
3. Machine learning (ML) techniques, including feature selection (FS) and feature learning (FL), have been applied to GT modeling to address challenges such as data scarcity and complex system behavior. These techniques have shown promise in fault diagnosis, prognosis, simulation, and design space exploration for GTs.
The article titled "Feature selection and feature learning in machine learning applications for gas turbines: A review" provides an overview of the use of machine learning (ML) techniques in gas turbine (GT) modeling, with a focus on feature selection (FS) and feature learning (FL). While the article offers valuable insights into the application of ML in GT modeling, there are several potential biases and limitations that need to be considered.
One potential bias is the limited scope of the review. The authors only include articles that emphasize FS and FL techniques in ML applications for GTs. This narrow focus may result in a biased representation of the overall field of ML-based GT modeling. Other important aspects, such as data preprocessing, model evaluation, and interpretability, are not adequately addressed in this review.
Another limitation is the lack of critical analysis and evaluation of the reviewed studies. The article mainly summarizes the findings and methodologies used in previous research without providing a comprehensive assessment of their strengths and weaknesses. This omission makes it difficult for readers to evaluate the reliability and applicability of the reviewed techniques.
Furthermore, there is a lack of discussion on potential risks and limitations associated with ML-based GT modeling. For example, ML models are often criticized for their black-box nature, making it challenging to interpret their decisions or identify potential biases in their predictions. The article does not address these concerns or provide guidance on how to mitigate them.
Additionally, there is a lack of exploration of counterarguments or alternative approaches to ML-based GT modeling. The article presents ML as the dominant approach without discussing potential drawbacks or limitations compared to traditional analytical methods or physics-based models. This one-sided reporting may lead readers to believe that ML is always superior to other approaches, which may not be true in all cases.
Moreover, there is a promotional tone throughout the article, particularly when discussing deep learning (DL) techniques. While DL has shown promise in various domains, including image recognition and natural language processing, its applicability and performance in GT modeling may be more limited. The article does not provide a balanced assessment of the strengths and weaknesses of DL in this specific context.
In conclusion, while the article provides a useful overview of ML applications in GT modeling, it has several limitations and biases that need to be considered. The narrow scope, lack of critical analysis, omission of potential risks, one-sided reporting, and promotional tone all contribute to a less comprehensive and balanced review. Readers should approach the findings with caution and seek additional sources for a more complete understanding of ML-based GT modeling.