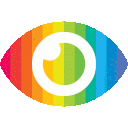
1. MRI tissue segmentation and classification is challenging due to a lack of signal intensity standardization.
2. The proposed method integrates quantitative MRI values (T1 and T2 relaxation times) and machine learning (Support Vector Machine) to segment and classify pelvic tissues.
3. The SVM classification accuracy was excellent for prostate, fat, muscle, bone marrow, and bladder, but not always successful for air within the rectum due to lack of training data.
作为一篇医学研究文章,该文的内容相对客观,但也存在一些潜在的偏见和局限性。首先,文章只针对男性进行了研究,因此不能完全代表女性的情况。其次,文章没有提及可能存在的风险和副作用,例如MRI扫描可能会对人体产生辐射影响。此外,在讨论中未探讨其他可能的方法或算法来解决MRI图像分割和分类问题。最后,该文并未提供足够的证据来支持所提出的算法在临床实践中的可行性和有效性。