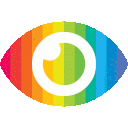
1. The Landing Approach Runway Detection (LARD) dataset is a collection of over 17K synthetic aerial images of runways, along with more than 1800 annotated pictures from real landing footage for comparison.
2. The dataset also includes a synthetic image generator based on Google Earth Studio, which can be used to generate high-quality synthetic pictures of airport runways with their metadata.
3. The LARD dataset is dedicated to the detection of runways during aircraft approach and landing phases, and it provides a valuable resource for vision-based landing systems.
The article titled "GitHub - deel-ai/LARD: A runway dataset and a generator of synthetic aerial images with automatic labeling" provides information about the Landing Approach Runway Detection (LARD) dataset and a synthetic image generator. While the article provides useful information about the dataset and its applications, there are some potential biases and missing points of consideration that should be addressed.
One potential bias in the article is the lack of discussion about the limitations of using synthetic images for training models. Synthetic images may not accurately represent real-world scenarios, leading to potential performance issues when deploying models trained on these images in real-life situations. It would have been helpful if the article had discussed any efforts made to address this limitation or provided examples of how the synthetic images compare to real-world data.
Additionally, the article does not provide enough evidence or details about the process used to annotate the real landing footage pictures included in the dataset. Without this information, it is difficult to assess the accuracy and reliability of these annotations. It would have been beneficial if the article had discussed any validation or quality control measures taken during the annotation process.
Furthermore, while the article mentions that over 1700 images were added to the test set of real images, it does not explain why these additional images were necessary or how they contribute to improving the dataset. Providing more context and justification for this addition would have strengthened the article's claims.
The article also lacks a discussion on potential risks or ethical considerations associated with using this dataset for vision-based landing systems. For example, it would have been valuable to explore any biases that may exist in both the synthetic and real image datasets and how these biases could impact model performance or safety in real-world applications.
In terms of promotional content, while it is understandable that this article aims to promote and provide information about LARD, there is a lack of critical analysis or exploration of alternative datasets or approaches. The article could have provided a more balanced perspective by discussing the strengths and weaknesses of LARD compared to other existing datasets or methods for runway detection.
Overall, while the article provides valuable information about the LARD dataset and its applications, there are potential biases, missing points of consideration, and unsupported claims that should be addressed to provide a more comprehensive and balanced analysis.