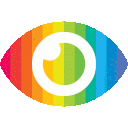
1. Automation and real-time reaction monitoring, combined with machine learning and artificial intelligence tools, are crucial in accelerating the identification of optimal reaction conditions and enabling error-free autonomous synthesis in chemical synthesis.
2. Data-rich experimentation (DRE) focuses on extracting real-time reaction progress data to provide a comprehensive understanding of reaction kinetics, intermediates, rate constants, and by-product reaction pathways. Automation plays a key role in making DRE approaches manageable and productive.
3. Machine learning and artificial intelligence tools enhance experimental data-driven workflows by predicting reaction conditions, reducing the number of experiments required for optimization, and improving the accuracy of outcome measurements. However, relying solely on existing literature data may lead to biased results, highlighting the need for systematic and standardized approaches to synthetic data analysis.
这篇文章探讨了化学合成中自动化、分析和人工智能的应用。然而,文章存在一些潜在的偏见和问题。
首先,文章过于强调数据丰富实验和跨学科方法在推动合成化学未来进展方面的重要性,但没有提及可能存在的局限性。例如,数据丰富实验可能需要大量时间和资源,并且并非所有实验都适合进行这种类型的实验。此外,跨学科方法可能导致信息传递和协作困难,因为不同领域的专家需要共同努力。
其次,文章引用了SARS-CoV-2疫情对药物开发的影响作为例子,但没有提供足够的证据来支持该例子。虽然确实有一些药物在短时间内得到开发并获得紧急使用授权,但这并不代表所有药物开发都可以如此迅速地完成。文章没有提及其他可能影响药物开发速度的因素,如资金、法规审批等。
此外,文章提到了自动化和机器学习在反应设计中的潜力,但没有详细说明如何应用这些技术以及它们可能面临的挑战。例如,在使用机器学习模型进行反应条件预测时,模型的准确性和可靠性是一个重要问题。文章没有提及如何解决这些问题。
最后,文章没有平衡地呈现双方观点。它过于强调数据丰富实验和自动化的优势,而忽视了传统实验方法的价值。虽然自动化和机器学习可以加快反应设计和优化过程,但它们并不是万能的解决方案,也不能完全取代人类的判断和经验。
综上所述,这篇文章在讨论自动化、分析和人工智能在化学合成中的应用时存在一些偏见和不足之处。读者需要对其中提出的主张保持批判性思维,并进一步探索相关领域的研究以获取更全面、客观的信息。