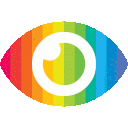
1. The accuracy of estimating population genetics parameters is highest when using a large sample size, even if the sequencing depth is low.
2. In some cases, a minimum sequencing depth of 2X is needed to accurately estimate allele frequencies and identify polymorphic sites.
3. Inferences of population structure are more accurate with very large sample sizes, even with extremely low sequencing depth.
The article titled "Assessing the Effect of Sequencing Depth and Sample Size in Population Genetics Inferences" by Matteo Fumagalli discusses the impact of sequencing depth and sample size on population genetics studies using Next-Generation Sequencing (NGS) technologies. The author conducted extensive simulations to evaluate the accuracy of estimating nucleotide diversity, detecting polymorphic sites, and predicting population structure under different experimental scenarios.
Overall, the article provides valuable insights into the optimal experimental design for population genetics studies using NGS data. However, there are a few potential biases and limitations in the article that should be considered.
Firstly, the article assumes that the cost of sequencing is proportional to the total sequencing depth. While this assumption may be reasonable in general, it does not take into account other factors that can affect the cost of sequencing, such as library preparation costs or data storage costs. Therefore, the conclusions drawn from this assumption may not be entirely accurate in real-world scenarios.
Secondly, the article focuses primarily on estimating nucleotide diversity and identifying polymorphic sites, but it does not discuss other important aspects of population genetics studies, such as detecting natural selection or inferring demographic history. These aspects could also be influenced by sequencing depth and sample size and should be considered in future research.
Additionally, the article does not explore potential trade-offs between sequencing depth and sample size. While larger sample sizes at lower sequencing depths may provide more accurate estimates of genetic variation, they may also result in a loss of power to detect rare variants or subtle population structure. It would be interesting to investigate these trade-offs further to determine the optimal balance between sequencing depth and sample size for different research questions.
Furthermore, the article does not discuss potential biases introduced by specific NGS technologies or data analysis methods. Different NGS platforms have different error rates and biases that can affect genotype calling accuracy. Similarly, different statistical methods for analyzing NGS data may have different assumptions and limitations. These factors should be taken into account when designing experiments and interpreting results.
Finally, the article does not address potential risks or limitations of using NGS data in population genetics studies. For example, sequencing errors or biases can lead to false positive or false negative results, which can affect the accuracy of population genetic inferences. It would be helpful to discuss these potential risks and provide recommendations for mitigating them.
In conclusion, while the article provides valuable insights into the impact of sequencing depth and sample size on population genetics studies using NGS data, there are some biases and limitations that should be considered. Future research should explore other aspects of population genetics studies, investigate trade-offs between sequencing depth and sample size, consider biases introduced by specific NGS technologies and data analysis methods, and address potential risks or limitations of using NGS data in population genetics research.