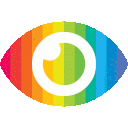
1. AtacWorks is a deep learning-based toolkit that denoises low-coverage or low-quality ATAC-seq data and improves the resolution of chromatin accessibility signal and identification of regulatory elements.
2. The neural network used in AtacWorks is based on the ResNet architecture, which allows for deeper and more accurate models to be trained.
3. AtacWorks can be applied to subsampled bulk ATAC-seq, single-cell ATAC-seq, and low-signal-to-noise ATAC-seq datasets, as well as adapted for cross-modality prediction of transcription factor footprints and ChIP-seq peaks from low-input ATAC-seq.
该文章介绍了一个基于深度学习的工具AtacWorks,用于提高ATAC-seq数据的质量和分辨率。然而,该文章存在一些潜在偏见和缺失的考虑点。
首先,该文章没有探讨深度学习模型可能存在的风险和局限性。例如,深度学习模型可能会出现过拟合问题,导致对新数据的泛化能力不足。此外,由于深度学习模型是黑盒子模型,其内部运作机制难以解释和理解,因此可能会引发一些伦理和法律问题。
其次,该文章没有平等地呈现双方。虽然该工具可以提高ATAC-seq数据的质量和分辨率,但并未探讨其对疾病诊断、治疗或基因编辑等方面的影响。此外,在使用该工具时需要注意到可能存在的误差和偏差,并进行相应的校正。
最后,该文章存在宣传内容和偏袒之嫌。尽管该工具可以提高ATAC-seq数据的质量和分辨率,但并未探讨其他类似工具或方法与其相比较时的优劣势。此外,在介绍该工具时也没有充分说明其开发者和资助方的背景和利益关系,可能存在潜在的偏袒。