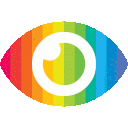
1. Highway traffic congestion is a major concern for mobility management, and data collection has been done for decades using various sensory systems.
2. Classification of highway traffic congestion has been conducted in different ways, mainly focused on either a theory-laden or data-driven approach.
3. Clustering analysis is unsupervised learning which aims to find an intrinsic partition of a dataset without any labelled items, and can be applied to explore potential partitions of traffic data.
The article "Feature extraction and clustering analysis of highway congestion" provides an overview of the challenges and opportunities in classifying highway traffic congestion patterns. The authors discuss the importance of collecting and managing large amounts of traffic data, as well as the need for efficient methods to classify and label this data. They review different approaches to classifying congestion patterns, including theory-laden and data-driven methods, and highlight the limitations of existing approaches.
The authors propose a new framework for classifying congested traffic patterns using unsupervised learning techniques. They describe two different feature extraction methods - point-based and area-based - which are used to represent traffic patterns as images. These images are then processed using computer vision techniques to extract representative features, which are used in a clustering algorithm to identify similar patterns in the dataset.
Overall, the article provides a comprehensive overview of the challenges and opportunities in classifying highway traffic congestion patterns. The authors provide a detailed description of their proposed framework, including its strengths and limitations. However, there are some potential biases in the article that should be noted.
Firstly, the authors focus primarily on unsupervised learning techniques for classifying congested traffic patterns. While these techniques have advantages over supervised learning methods (such as not requiring labeled data), they also have limitations in terms of accuracy and interpretability. It would be useful for the authors to acknowledge these limitations and discuss how they might be addressed in future research.
Secondly, while the authors provide a detailed description of their proposed feature extraction methods (point-based and area-based), they do not provide much detail on how these methods were developed or validated. It would be useful for them to provide more information on how these methods were tested on real-world datasets, as well as any limitations or potential biases associated with them.
Finally, while the authors acknowledge some potential risks associated with their proposed framework (such as inaccurate classification due to noisy data), they do not discuss other potential risks such as privacy concerns or unintended consequences of automated classification systems. It would be useful for them to address these issues more explicitly in future research.
In conclusion, while there are some potential biases in this article (such as a focus on unsupervised learning techniques), overall it provides a valuable contribution to our understanding of how machine learning can be used to classify congested traffic patterns. The proposed framework has potential applications in transportation planning and management, but further research is needed to validate its effectiveness and address any potential risks or limitations.