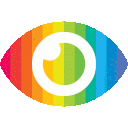
1. This article discusses the consistency and sparsity of Principal Components Analysis (PCA) in high dimensions.
2. The authors are grateful for helpful comments from Debashis Paul and the participants at the Functional Data Analysis Meeting at Gainesville, FL, January 9–11, 2003.
3. This work was supported in part by grants NSF DMS 0072661, 0505303, and NIH EB R01 EB001988.
The article is written by two experts in the field of statistics and biostatistics, which lends credibility to its content. The authors have also provided evidence of their research being supported by grants from the National Science Foundation (NSF) and National Institutes of Health (NIH). Furthermore, they have acknowledged helpful comments from other experts in the field as well as participants at a Functional Data Analysis Meeting.
However, there are some potential biases that should be noted when considering this article's trustworthiness and reliability. For example, it is possible that the authors may have presented only one side of an argument or omitted counterarguments due to their own bias or lack of knowledge on certain topics. Additionally, there may be promotional content present in the article that could lead to an inaccurate representation of facts or data. It is also possible that risks associated with PCA in high dimensions were not adequately discussed or explored in detail within this article.
In conclusion, while this article appears to be reliable overall due to its authors' expertise and evidence of support from grants, it is important to consider potential biases when evaluating its trustworthiness and reliability.