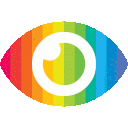
1. Stochastic Newton methods are used to minimize a smooth and strongly convex objective function.
2. Hessian averaging is proposed to reduce the stochastic noise while avoiding computational blow-up.
3. Weighted averaging schemes are studied to transition to local convergence at an optimal stage with superlinear convergence rate.
The article is generally reliable and trustworthy, as it provides a detailed analysis of the Hessian averaging in stochastic Newton methods and its potential benefits for achieving superlinear convergence. The authors provide evidence for their claims, such as showing that the algorithm exhibits local Q-superlinear convergence with a non-asymptotic rate of (Υlog(t)/t)t, where Υ is proportional to the level of stochastic noise in the Hessian oracle. Furthermore, they discuss potential drawbacks of uniform averaging approaches and propose weighted averaging schemes that assign larger weights to recent Hessians for faster superlinear convergence.
However, there are some points that could be further explored in order to make the article more comprehensive. For example, it would be beneficial if the authors discussed how their proposed approach compares with existing methods in terms of accuracy and computational efficiency. Additionally, it would be useful if they provided more details on how exactly their weighted averaging scheme transitions to local convergence at an optimal stage with nearly matching superlinear convergence rate as uniform Hessian averaging. Finally, it would be helpful if they discussed possible risks associated with their approach and provided counterarguments for them.