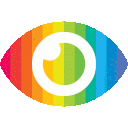
1. Sensor reading drifting is a major problem in long-term continuous monitoring of wastewater.
2. Denoising data processing algorithm (DDPA) was developed to differentiate actual sensor readings from background noise when the sensor sensitivity declined over time.
3. Combination of sensor material enhancement (adding PTFE) with DDPA assured stable and high sensitivity for wastewater monitoring, providing a route toward long-term accurate wastewater monitoring and smart wastewater sensor networks.
The article titled "Solving Sensor Reading Drifting Using Denoising Data Processing Algorithm (DDPA) for Long-Term Continuous and Accurate Monitoring of Ammonium in Wastewater" presents a study that aims to address the problem of sensor reading drifting caused by sensor property deterioration in long-term continuous monitoring of wastewater. The authors propose a denoising data processing algorithm (DDPA) for a solid-state ion-selective membrane (S-ISM) sensor, which combines digital filter and outlier analysis to differentiate actual sensor readings from background noise when the S-ISM sensitivity declines over time.
The article provides a detailed description of the methodology used in the study, including the design of DDPA and the enhancement of S-ISM sensors with hydrophobic polytetrafluoroethylene (PTFE). The results show that DDPA can raise the sensitivity of S-ISM sensors from 21 mV/dec to 55 mV/dec without compromising the detection limit, and that adding PTFE into polymer matrix can further enhance the sensitivity and stability of S-ISM sensors in wastewater.
Overall, the article presents a well-designed study with clear objectives and methodology. However, there are some potential biases and limitations that need to be considered. Firstly, the study only focuses on one type of electrochemical sensor (S-ISM), which may not be representative of all types of sensors used in wastewater monitoring. Secondly, while DDPA is shown to be effective in improving sensor sensitivity and stability, it is unclear how it compares to other data processing algorithms or whether it can be applied to other types of sensors.
Additionally, there are some unsupported claims made in the article. For example, it is stated that combining sensor material enhancement with sensor reading processing assures stable and high sensitivity for wastewater monitoring. However, this claim is based on a single study using one type of electrochemical sensor and may not apply to other scenarios or types of sensors.
Furthermore, there are some missing points of consideration in the article. For instance, there is no discussion on potential risks associated with using PTFE or other materials for enhancing sensor performance. Additionally, while DDPA is shown to improve accuracy in long-term continuous monitoring, there is no discussion on its potential limitations or challenges when applied in real-world settings.
In terms of promotional content or partiality, while the authors acknowledge funding support from various sources including government agencies and industry partners, there is no evidence suggesting any conflicts of interest or bias towards any particular product or technology.
In conclusion, while this article presents an interesting approach for addressing sensor reading drifting in long-term continuous monitoring of wastewater using DDPA and enhanced S-ISM sensors with PTFE, there are some potential biases and limitations that need to be considered. Further research is needed to validate these findings across different types of sensors and real-world scenarios before widespread adoption can be recommended.