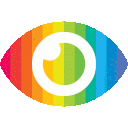
1. The Internet of Things (IoT) market is rapidly growing, with an estimated worth of up to $42 billion by 2026. However, IoT devices are vulnerable to cyber attacks due to flaws such as weak passwords, outdated firmware, and lack of encryption algorithms.
2. Denial of Service (DoS) attacks continue to be a significant concern for home-based IoT devices, as they can disrupt network services and compromise user data. In this study, researchers conducted DoS attacks on four different IoT devices to understand their vulnerabilities from a network level.
3. The study proposes an effective Intrusion Detection technique using a combination of machine learning classifiers and deep learning to detect and mitigate DoS attacks. The findings also highlight the need for improved security protocols and design implications to address critical security flaws in home-based IoT devices.
The article "Exploring The Security Issues in Home-Based IoT Devices Through Denial of Service Attacks" provides a comprehensive overview of the vulnerabilities and risks associated with IoT devices. The authors conducted a Denial of Service (DoS) attack on four different IoT devices to understand their vulnerabilities from a network level. They also proposed an effective Intrusion Detection technique using machine learning classifiers and deep learning.
Overall, the article provides valuable insights into the security issues surrounding IoT devices. However, there are some potential biases and limitations that need to be considered. For example, the study was conducted in a lab environment, which may not accurately reflect real-world scenarios. Additionally, the authors only focused on DoS attacks and did not explore other types of cyber-attacks that could target IoT devices.
Furthermore, while the article highlights the importance of securing IoT devices, it does not provide practical solutions for consumers to protect themselves from cyber-attacks. It would have been helpful if the authors had provided recommendations or guidelines for users to secure their IoT devices.
Another limitation is that the article does not address the ethical implications of using machine learning classifiers and deep learning for intrusion detection. There is a risk that these techniques could be used to invade users' privacy by monitoring their behavior without their consent.
In terms of promotional content, while the article does mention specific brands such as Amazon Echo and Phillips Hue Light as examples of popular IoT devices, it does not appear to promote any particular product or company.
Overall, while there are some limitations and potential biases in this article, it provides valuable insights into the security issues surrounding IoT devices and proposes an effective solution for detecting DoS attacks. However, further research is needed to address other types of cyber-attacks and provide practical solutions for consumers to secure their IoT devices.