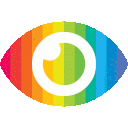
1. Researchers used quantitative CT imaging and machine learning to differentiate between chronic obstructive pulmonary disease (COPD) and asthma patients.
2. A subset of seven CT features, including low-attenuating area, airway perimeters and areas, and total airway count, were found to be effective in distinguishing between COPD and asthma.
3. Machine learning achieved moderate-to-high accuracy in differentiating COPD and asthma, with reduced CT emphysema and total airway count playing a significant role in distinguishing between the two conditions.
The article titled "Differentiating COPD and asthma using quantitative CT imaging and machine learning" presents a study that aims to determine the optimal subset of CT imaging features for differentiating between chronic obstructive pulmonary disease (COPD) and asthma using machine learning techniques. While the study provides valuable insights into the potential use of CT imaging and machine learning in distinguishing between these two respiratory conditions, there are several aspects that require critical analysis.
One potential bias in the article is the selection of participants from a single hospital, Heidelberg University Hospital in Germany. This may limit the generalizability of the findings to other populations or healthcare settings. Additionally, the sample size of 95 participants (48 with COPD and 47 with asthma) is relatively small, which could affect the statistical power and reliability of the results.
The article claims that COPD and asthma can be differentiated with moderate-to-high accuracy using a subset of only seven CT features. However, it does not provide detailed information on how these features were selected or validated. The methodology section mentions "hybrid feature selection," but it does not explain this approach or provide any references to support its effectiveness.
Furthermore, while the study identifies specific CT features that are important for differentiating between COPD and asthma, it does not explore potential confounding factors or consider other clinical variables that could influence these findings. For example, smoking history, environmental exposures, and comorbidities could impact both CT imaging features and disease classification.
The article also lacks discussion on potential limitations or risks associated with using machine learning algorithms for disease classification. Machine learning models heavily rely on training data, which may introduce biases if not properly representative of the target population. Additionally, overfitting or underfitting issues can occur if models are not adequately validated on independent datasets.
Another limitation is that the article does not present any counterarguments or alternative approaches to differentiating between COPD and asthma. It would be valuable to discuss how other diagnostic methods, such as pulmonary function tests or biomarkers, compare to CT imaging and machine learning in terms of accuracy and clinical utility.
The article does not provide a balanced presentation of both the benefits and limitations of using CT imaging and machine learning for disease differentiation. It primarily focuses on the positive findings and highlights the potential of these techniques without adequately addressing their limitations or potential risks.
In conclusion, while the study presented in the article offers insights into the use of CT imaging and machine learning for differentiating between COPD and asthma, there are several limitations and biases that need to be considered. The small sample size, single-center recruitment, lack of detailed methodology explanation, absence of alternative approaches, and limited discussion on potential risks all contribute to a less comprehensive analysis. Further research with larger sample sizes, diverse populations, and rigorous validation is needed to fully assess the accuracy and clinical utility of these techniques in distinguishing between COPD and asthma.