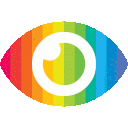
1. The healthcare industry is facing challenges in managing electronic records, integrating data, and making accurate diagnoses and disease predictions.
2. Deep learning and predictive analytics are emerging as transformative tools in healthcare, offering techniques to address these challenges and enable proactive and preventative treatment options.
3. Deep learning is being applied in various areas of healthcare, including drug discovery, medical imaging analysis, insurance fraud detection, Alzheimer's disease diagnosis, and understanding genomes.
The article titled "Deep Learning for Predictive Analytics in Healthcare" provides an overview of the role of deep learning in healthcare data analysis and predictive analytics. While the article offers valuable insights into the potential applications of deep learning in healthcare, there are several areas where a critical analysis is warranted.
Firstly, the article highlights the challenges faced by the healthcare industry in areas such as electronic record management, data integration, and computer-aided diagnoses. However, it does not provide sufficient evidence or examples to support these claims. Without concrete examples or references to studies or reports, it is difficult to assess the extent of these challenges and their impact on healthcare outcomes.
Additionally, the article mentions that deep learning offers a wide range of tools and techniques to address these challenges but does not provide specific details about these tools or techniques. It would have been helpful to include examples of deep learning algorithms or frameworks that have been successfully applied in healthcare settings.
Furthermore, the article focuses primarily on the benefits and potential applications of deep learning in healthcare without adequately addressing potential risks or limitations. Deep learning algorithms rely heavily on large volumes of data, which raises concerns about privacy and security. The article does not discuss how these issues are addressed or mitigated in practice.
Moreover, while the article briefly mentions that predictive models have limited use in clinical practice, it does not explore the reasons behind this limitation. Factors such as lack of integration with existing clinical workflows and difficulties in adapting models from study populations to local populations are important considerations that should have been discussed further.
Another aspect that deserves attention is potential biases in the article. The article seems to present a somewhat one-sided view of deep learning as a transformative tool without adequately discussing alternative approaches or considering potential drawbacks. This could be seen as promotional content for deep learning technologies without providing a balanced perspective.
In conclusion, while the article provides an introduction to deep learning for predictive analytics in healthcare, it lacks sufficient evidence and critical analysis. It would have been beneficial to include more concrete examples, address potential risks and limitations, and provide a balanced view of deep learning in healthcare.