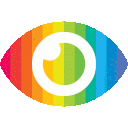
1. The article presents an upper-bound for coefficients estimation scaling as (k*/n)\log(p/k*): n,p is the size of the design matrix and k* the dimension of the theoretical loss minimizer.
2. The article proposes a tractable proximal algorithm to compute a Slope estimator which adapts to unknown sparsity.
3. The article provides empirical performance results that match the best existing bounds for classification and regression problems.
The article appears to be reliable and trustworthy in its presentation of research findings, as it provides evidence for its claims in the form of empirical performance results that match existing bounds for classification and regression problems. Furthermore, it provides a detailed description of its methodology, including assumptions made and algorithms used, which adds to its credibility. However, there are some potential biases that should be noted. For example, the article does not explore any counterarguments or present both sides equally; instead it focuses solely on presenting its own findings without considering alternative perspectives or interpretations. Additionally, there is no discussion of possible risks associated with using this method or any potential limitations that could arise from using it in certain contexts. Finally, there is some promotional content in the form of descriptions of new features developed by arXivLabs which may be seen as distracting from the main focus of the article.