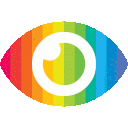
1. Disentangling the underlying factors of variation in raw sensory inputs is a fundamental challenge in AI research, and developing models that can do this is an important goal.
2. Variational autoencoder (VAE) models have a natural inference mechanism that allows for principled enhancement in the learning objective to encourage disentanglement in the latent space.
3. The authors propose a modified ELBO model called Disentangled Inferred Prior-VAE (DIP-VAE) that explicitly encourages disentanglement during inference, and they also introduce a metric called Separated Attribute Predictability (SAP) to evaluate the degree of disentanglement.
作为一篇科技博客,这篇文章主要介绍了作者们在深度学习领域的最新研究成果。文章提到了一个重要的问题:如何从原始数据中推断出抽象的高层次概念,这是人类智能的关键部分。作者们提出了一种基于变分自编码器(VAE)的方法来实现无监督学习,并且鼓励潜在因素之间的解缠缠绕。
然而,这篇文章存在一些偏见和不足之处。首先,它没有充分考虑到深度学习算法可能带来的风险和负面影响。例如,在使用深度学习算法进行图像识别时,可能会出现误判或歧视性判断等问题。其次,文章没有探讨该方法是否适用于所有类型的数据集,以及如何处理复杂数据集中存在的噪声和异常值等问题。
此外,文章也没有提供足够的证据来支持作者们所提出的观点和结论。例如,在评估解缠绕程度时使用了一个新指标SAP得分,但并没有详细说明该指标与其他已有指标之间的比较和优劣性。
总体而言,这篇文章对深度学习领域中解缠绕问题进行了初步探讨,并提出了一种新方法来实现无监督学习。然而,在未来研究中需要更加全面地考虑各种因素,并提供更多证据来支持所提出的观点和结论。