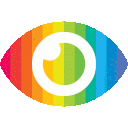
1. The wavelet threshold-denoising method is adopted to preprocess training data for stock price prediction, as deep neural networks trained by original data fail to accurately predict due to high noise in financial data.
2. A novel multioptimal combination wavelet transform (MOCWT) method is proposed, which outperforms traditional methods in terms of prediction accuracy.
3. The LSTM model based on the concept of RNN is used for stock prediction applications, with its "gate recurrent" structure allowing it to remember effective information while forgetting useless information.
The article "LSTM with Wavelet Transform Based Data Preprocessing for Stock Price Prediction" discusses the use of deep learning methods, specifically LSTM neural networks, for stock price prediction. The article highlights the challenges of predicting stock prices due to the high noise in financial data and proposes a wavelet threshold-denoising method to preprocess training data. The article also introduces a new multioptimal combination wavelet transform (MOCWT) method that outperforms traditional methods in terms of prediction accuracy.
Overall, the article provides a comprehensive overview of the current state-of-the-art in stock price prediction using deep learning methods. However, there are some potential biases and limitations to consider.
One potential bias is that the article focuses solely on deep learning methods and does not explore other approaches to stock price prediction, such as traditional statistical models or economic methods. While deep learning has shown promising results in this field, it is important to consider all available options when making investment decisions.
Another limitation is that the article does not provide a detailed explanation of how the wavelet threshold-denoising method works or how it improves prediction accuracy. Without this information, it is difficult to assess the validity of this approach.
Additionally, while the MOCWT method is shown to outperform traditional methods in terms of prediction accuracy, there is no discussion of potential risks or drawbacks associated with this approach. It would be helpful to explore any limitations or potential downsides to using this method before implementing it in real-world applications.
Finally, while the article presents evidence supporting its claims about the effectiveness of LSTM neural networks and wavelet preprocessing for stock price prediction, there may be unexplored counterarguments or missing evidence that could challenge these claims. It would be beneficial for future research to address any gaps in knowledge and provide a more complete understanding of these techniques' effectiveness.