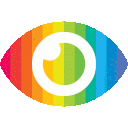
1. This article reviews recent developments in turbulence modeling, with a focus on using data to reduce model uncertainties.
2. It discusses key principles, achievements, and challenges related to bounding uncertainties in RANS models via physical constraints, statistical inference for characterizing model coefficients and estimating discrepancy, and machine learning for improving turbulence models.
3. A central perspective advocated in this review is that by exploiting foundational knowledge in turbulence modeling and physical constraints, researchers can use data-driven approaches to yield useful predictive models.
The article “Turbulence Modeling in the Age of Data” provides an overview of recent developments in turbulence modeling with a focus on using data to reduce model uncertainties. The authors provide a comprehensive review of the current state of the art in this field, discussing key principles, achievements, and challenges related to bounding uncertainties in RANS models via physical constraints, statistical inference for characterizing model coefficients and estimating discrepancy, and machine learning for improving turbulence models.
The article is generally well written and provides a thorough overview of the topic at hand. However, there are some potential biases that should be noted. For example, the authors do not discuss any potential risks associated with using data-driven approaches or any counterarguments that could be made against them. Additionally, they do not present both sides equally when discussing the advantages of using data-driven approaches versus traditional methods; instead they focus solely on the benefits of using such approaches without exploring any potential drawbacks or limitations. Furthermore, there is no evidence provided to support some of the claims made throughout the article; instead it relies heavily on opinion rather than facts or research findings.
In conclusion, while this article provides an informative overview of recent developments in turbulence modeling with a focus on using data to reduce model uncertainties, it does contain some potential biases which should be taken into consideration when evaluating its trustworthiness and reliability.