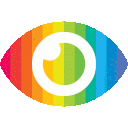
1. The increasing availability of remote sensing data from multiple sources has led to a need for multimodal data classification methods.
2. A deep learning-based framework called the Cross-Channel Reconstruction Network (CCR-Net) is proposed for multimodal remote sensing data classification.
3. Experiments on two multimodal datasets demonstrate the effectiveness and superiority of the proposed method compared to state-of-the-art methods.
The article titled "Convolutional Neural Networks for Multimodal Remote Sensing Data Classification" presents a new framework for multimodal remote sensing data classification using convolutional neural networks (CNNs) and an advanced cross-channel reconstruction module called CCR-Net. The authors claim that their proposed method outperforms several state-of-the-art multimodal RS data classification methods.
Overall, the article provides a detailed description of the proposed method and its experimental results on two multimodal RS datasets. However, there are some potential biases and limitations in the article that need to be considered.
Firstly, the article focuses only on the proposed method and does not provide a comprehensive comparison with other existing methods. While the authors claim that their method outperforms several state-of-the-art methods, it is unclear how it compares to other recent approaches in this field.
Secondly, the article does not discuss any potential limitations or risks associated with using deep learning-based methods for remote sensing data classification. For example, deep learning models require large amounts of labeled training data, which may not always be available in remote sensing applications. Additionally, deep learning models can be computationally expensive and may require specialized hardware for efficient training and inference.
Thirdly, while the authors provide some details about their experimental setup and results, they do not discuss any potential sources of bias or uncertainty in their experiments. For example, it is unclear how they selected their training and test datasets or how they ensured that their results were statistically significant.
Finally, the article contains some promotional content regarding the availability of codes for reproducibility purposes. While this is certainly a positive aspect of the research, it should not overshadow any potential limitations or biases in the study itself.
In conclusion, while the article presents an interesting new approach for multimodal remote sensing data classification using CNNs and CCR-Net, there are some potential biases and limitations that need to be considered when interpreting its results. Future research should aim to address these issues by providing more comprehensive comparisons with existing methods, discussing potential limitations and risks associated with deep learning-based approaches for remote sensing data analysis, and ensuring that experimental results are robust to sources of bias or uncertainty.