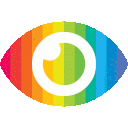
1. The state of health (SOH) of lithium-ion batteries is highly dependent on usage, stress, and environmental conditions, making it difficult to accurately forecast in real-world scenarios.
2. Current models for SOH forecasting are limited by unrealistic assumptions and a lack of consideration for factors such as calendar ageing and varying charging currents.
3. A proposed method for SOH forecasting addresses these challenges by extracting suitable stressor data, using machine learning regression models, and considering the temporal aggregation of battery operation time and signal ranges.
The article titled "State of health forecasting of Lithium-ion batteries applicable in real-world operational conditions" provides an overview of the current state of research on battery state of health (SOH) forecasting, with a focus on lithium-ion batteries used in electric vehicles. The article highlights the importance of accurate SOH forecasting for fleet managers and battery designers, and outlines the challenges associated with developing models that can accurately predict battery ageing based on real-world usage data.
Overall, the article provides a comprehensive overview of the topic and presents a clear argument for the need to develop more accurate SOH forecasting models. However, there are several potential biases and limitations to consider when evaluating the article's claims.
One potential bias is that the article focuses exclusively on lithium-ion batteries, which are currently the most widely used type of battery in electric vehicles. While this is understandable given their prevalence in the market, it is important to note that other types of batteries may have different ageing characteristics and require different modelling approaches.
Another potential bias is that the article assumes that SOH forecasting is necessary for all electric vehicle fleets, regardless of their size or usage patterns. While this may be true for large fleets with high levels of usage, smaller fleets or individual vehicle owners may not require such detailed forecasting capabilities.
Additionally, while the article acknowledges some of the challenges associated with developing accurate SOH forecasting models based on real-world usage data, it does not explore potential counterarguments or alternative approaches to addressing these challenges. For example, it may be possible to develop more accurate models by combining laboratory data with real-world usage data or by using machine learning algorithms to identify patterns in large datasets.
Finally, while the article notes some potential risks associated with inaccurate SOH forecasting (such as premature battery failure), it does not provide a comprehensive analysis of these risks or explore potential solutions for mitigating them.
In conclusion, while "State of health forecasting of Lithium-ion batteries applicable in real-world operational conditions" provides a useful overview of current research on SOH forecasting for lithium-ion batteries used in electric vehicles, readers should be aware of its potential biases and limitations when evaluating its claims.