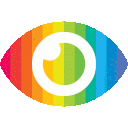
1. Spatial transcriptomics provides important insights into biological function and pathology, but current methods have limitations in resolution.
2. BayesSpace is a computational method that uses neighborhood structure to increase resolution to the subspot level, approaching single-cell resolution with the Visium platform.
3. BayesSpace enables spatial clustering by modeling a low-dimensional representation of the gene expression matrix and encouraging neighboring spots to belong to the same cluster via a spatial prior, improving identification of spatially distributed tissue domains.
作为一篇科学论文,该文章并没有明显的偏见或宣传内容。然而,它可能存在一些片面报道和缺失的考虑点。
首先,文章提到了现有的空间基因表达数据分析方法的限制,包括分辨率低和需要新的统计方法等。然而,它没有探讨这些限制对研究结果的影响以及如何解决这些问题。
其次,文章强调了BayesSpace方法可以在不需要单细胞数据的情况下实现高分辨率建模,并且可以推断亚斑点的空间排列。然而,它没有探讨BayesSpace方法是否适用于所有类型的生物组织和样本,并且是否存在其他因素会影响其准确性和可靠性。
此外,在介绍现有空间基因表达数据分析方法时,文章只提到了少数几种方法,并未全面涵盖所有可用技术。这可能导致读者对该领域中其他重要技术和进展的认识不足。
最后,在介绍BayesSpace方法时,文章没有探讨该方法可能存在的风险或局限性。例如,使用贝叶斯统计学进行超分辨率图像处理可能会导致过度平滑或过度拟合等问题。
总之,尽管该文章并未明显偏袒任何一方或宣传特定观点,但仍存在一些片面报道、缺失考虑点和未探索反驳等问题。