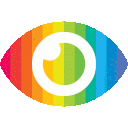
1. The public mood states derived from large-scale Twitter feeds are correlated to the value of the Dow Jones Industrial Average (DJIA) over time.
2. Certain dimensions of public mood states, such as Calm and Happiness, increase the accuracy of a Self-Organizing Fuzzy Neural Network model in predicting up and down changes in DJIA closing values to 87.6%.
3. The accuracy of DJIA predictions can be significantly improved by the inclusion of specific public mood dimensions but not others, with an accuracy of 86.7% in predicting daily up and down changes in the closing values of the DJIA and a reduction of the Mean Average Percentage Error (MAPE) by more than 6%.
The article "Twitter mood predicts the stock market" explores the relationship between public mood states and the Dow Jones Industrial Average (DJIA) using Twitter feeds. The authors use two mood tracking tools, OpinionFinder and Google-Profile of Mood States (GPOMS), to measure positive vs. negative mood and six dimensions of mood, respectively. They find that certain dimensions of public mood states, particularly Calm and Happiness as measured by GPOMS, can predict changes in DJIA closing values with an accuracy of 87.6%.
While the study presents interesting findings, there are several potential biases and limitations to consider. Firstly, the study only analyzes tweets from a specific time period in 2008, which may not be representative of current social media trends or public sentiment. Additionally, the study only focuses on one stock market index (DJIA), which may not be reflective of broader economic trends.
Furthermore, the study relies heavily on sentiment analysis tools that may not accurately capture nuanced emotions or sarcasm in tweets. This could lead to inaccurate assessments of public mood states and their relationship to stock market performance.
Another limitation is that the study does not account for other factors that may influence stock market performance, such as political events or economic indicators. While the authors attempt to control for some external factors by cross-validating their results against public responses to the presidential election and Thanksgiving day in 2008, there may be other confounding variables at play.
Finally, it is important to note that while predicting stock market performance based on public sentiment may have practical applications for investors, it also raises ethical concerns about manipulating public opinion for financial gain.
Overall, while this study provides interesting insights into the relationship between social media and stock market performance, it is important to approach its findings with caution and consider potential biases and limitations.