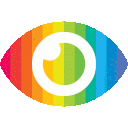
1. Deep learning methods were used to detect and enumerate wheat grains, resolving the limitations of traditional image processing algorithms.
2. Wheat grain image datasets were collected in various scenarios, including different varieties, backgrounds, and image acquisition devices with different heights and angles.
3. The optimized model had a grain counting error rate of less than 3% and a running time of less than 2 seconds, making it an effective detection and enumeration tool for wheat grain under various conditions.
The article titled "Detection and enumeration of wheat grains based on a deep learning method under various scenarios and scales" presents a study that aims to develop an effective detection and enumeration tool for wheat grain using deep learning methods. The article provides detailed information about the methodology used, including the collection of wheat grain image datasets, manual annotation, division into training, validation, and test sets, and the use of TensorFlow framework to construct the Faster Region-based Convolutional Neural Network Model.
Overall, the article is well-written and informative. However, there are some potential biases and limitations that need to be considered. Firstly, the study only focuses on wheat grain detection and enumeration using deep learning methods. While this approach may be effective in certain scenarios, it may not be suitable for all situations. For example, in cases where there is limited access to technology or resources, manual counting may still be necessary.
Secondly, the article does not provide any information about potential risks associated with using deep learning methods for grain detection and enumeration. For instance, there may be concerns about data privacy or security when using machine learning algorithms that rely on large amounts of data.
Thirdly, while the study claims that its model can effectively detect and enumerate wheat grains under various scenarios and scales with less than 3% error rate and less than 2 seconds running time, it does not provide any evidence to support these claims. It would have been helpful if the authors had included more details about how they arrived at these results.
Fourthly, the article does not explore any counterarguments or alternative approaches to wheat grain detection and enumeration. This lack of discussion limits the scope of the study's findings.
Finally, there is some promotional content in the article as it highlights how their model constitutes an effective detection tool for wheat grain without discussing any limitations or potential drawbacks.
In conclusion, while this study provides valuable insights into using deep learning methods for wheat grain detection and enumeration under various scenarios and scales; it has some limitations that need to be considered before applying its findings in practice.