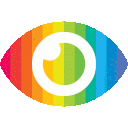
1. Step count is a well-known predictor of long-term morbidity and mortality, but a lack of methodological standards and clinical validation remains a major impediment to its acceptance as a valid clinical endpoint.
2. Previous step-counting algorithms have often been device-specific and may not be widely available, limiting their suitability in clinical scenarios.
3. Deep learning models offer superior predictive power and classification accuracy for step detection, but overfitting can be a problem with small datasets. Domain adaptation can address this issue and provide highly accurate step counts for both generalization and personalization approaches.
The article "Accurate Step Count with Generalized and Personalized Deep Learning on Accelerometer Data" discusses the importance of step count as a measure of physical activity and its potential as a clinical endpoint or biomarker. The authors highlight the lack of methodological standards and clinical validation for step-counting algorithms, which limits their suitability in clinical scenarios. They also note that previous studies have mainly focused on device-specific algorithms and often employ sensor modalities that may not be widely available.
To address these limitations, the authors trained neural network models on publicly available data and tested them on an independent cohort using two approaches: generalization and personalization. The best models exhibited highly accurate step-count estimates for both approaches, demonstrating the possibility of developing device-agnostic, accelerometer-only algorithms that provide reliable mobility endpoints.
Overall, the article provides valuable insights into the potential of deep learning models for step detection and highlights the need for standardized and validated algorithms in clinical settings. However, there are some potential biases and limitations to consider.
One limitation is that the study only used accelerometer data, which may not capture all types of physical activity accurately. For example, activities such as cycling or swimming may not be detected by accelerometers alone. Additionally, the study did not explore potential counterarguments or limitations to deep learning models for step detection.
Another potential bias is that the study was conducted using publicly available data from healthy subjects, which may not generalize to other populations or clinical scenarios. The authors acknowledge this limitation but do not provide further discussion on how it may impact their findings.
Finally, while the article does not contain overtly promotional content, it does focus heavily on the potential benefits of deep learning models for step detection without fully exploring potential risks or limitations. This could potentially lead readers to overestimate the effectiveness of these models in real-world settings.
In conclusion, while "Accurate Step Count with Generalized and Personalized Deep Learning on Accelerometer Data" provides valuable insights into the potential of deep learning models for step detection, readers should approach its findings with caution and consider potential biases and limitations when interpreting its results.