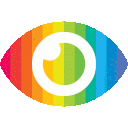
1. WiFi indoor positioning technology is widely studied due to the extensive deployment of wireless networks in indoor environments and the fact that most mobile devices are equipped with built-in WiFi modules.
2. The weighted K-nearest neighbors (WKNN) algorithm is a prevalent method for indoor WiFi positioning based on location fingerprinting, but it suffers from challenges such as a fixed K value and susceptibility to incorrect reference point matching.
3. The proposed static continuous statistical characteristics-soft range limited-self-adaptive WKNN (SCSC-SRL-SAWKNN) algorithm takes into account location tracking in both motion and stationary states, employs SAWKNN and SRL-KNN algorithms to achieve the best positioning accuracy, and uses Kalman filter to generate the location trajectory. It outperforms traditional WKNN, SAWKNN, and SRL-KNN techniques in terms of localization accuracy and location trajectory.
作为一篇科技论文,该文章并没有明显的偏见或宣传内容。然而,它可能存在一些片面报道和缺失的考虑点。
首先,文章强调了WiFi室内定位技术的优势,但没有提及其潜在的隐私风险。由于WiFi信号可以穿透墙壁,因此可能会泄露用户的位置信息。这是一个重要的问题,需要在使用WiFi室内定位技术时加以考虑。
其次,在讨论现有算法的挑战时,文章只提到了WKNN算法面临的问题,并未探讨其他算法可能存在的局限性。这可能导致读者对WKNN算法过于批判或忽略其他算法的优点。
此外,在介绍作者提出的新算法时,文章没有提供足够的证据来支持其声称具有更高精度和更好轨迹跟踪能力。这使得读者难以评估该算法是否真正有效。
最后,在讨论WiFi室内定位技术时,文章没有涉及到与其他定位技术相比所需成本和可行性等方面进行比较。这可能导致读者对该技术过于乐观或忽略了其他选择。
总之,尽管该文章并未明显偏袒或宣传,但仍存在一些片面报道和缺失的考虑点。读者需要对其内容进行更全面的评估,并考虑其他可能存在的风险和选择。