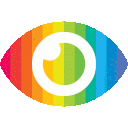
1. Accurate and reliable forecasting models for electricity demand are critical in addressing energy sustainability challenges for the National Electricity Market (NEM) in Australia.
2. Data-driven techniques for forecasting short-term electricity demand data are adopted using Multivariate Adaptive Regression Spline (MARS), Support Vector Regression (SVR), and Autoregressive Integrated Moving Average (ARIMA) models.
3. The MARS and SVR models can be considered more suitable for short-term electricity demand forecasting in Queensland, Australia, when compared to the ARIMA model.
The article titled "Short-term electricity demand forecasting with MARS, SVR and ARIMA models using aggregated demand data in Queensland, Australia" provides an overview of data-driven techniques for forecasting short-term electricity demand in Queensland, Australia. The study compares the Multivariate Adaptive Regression Spline (MARS), Support Vector Regression (SVR), and Autoregressive Integrated Moving Average (ARIMA) models to determine their accuracy in predicting electricity demand.
The article is well-written and provides a comprehensive overview of the study's methodology and results. However, there are some potential biases and limitations that should be considered when interpreting the findings.
One potential bias is that the study only focuses on short-term forecasting horizons of 0.5 hours, 1 hour, and 24 hours. This may not be sufficient for long-term planning purposes or for predicting changes in energy consumption patterns over time. Additionally, the study only uses aggregated demand data rather than individual consumer data, which may limit its accuracy in predicting specific energy usage patterns.
Another limitation is that the study only focuses on one region of Australia (Queensland) and may not be generalizable to other regions or countries with different energy consumption patterns or regulatory frameworks.
Furthermore, while the article notes that accurate electricity demand forecasting is critical for addressing energy sustainability challenges, it does not explore potential risks associated with inaccurate forecasting such as overinvestment in infrastructure or underinvestment leading to blackouts or brownouts.
Overall, while the article provides valuable insights into data-driven techniques for short-term electricity demand forecasting in Queensland, it is important to consider its limitations and potential biases when interpreting its findings.