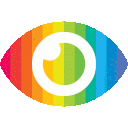
1. This study compared the performance of Gradient Boosting Decision Trees (GBDT), Artificial Neural Networks (ANN) and Random Forests (RF) in modeling Land Use Change (LUC) in the Seoul Metropolitan Area.
2. Results showed that GBDT and RF had higher predictive power than ANN, indicating that tree-based ensemble methods are an effective LUC prediction technique.
3. The results from GBDT showed that distance to existing residential land was the most important predictor of urban land use change, accounting for 30.4% of relative importance, followed by proximity to industrial and public lands which accounted for 32.3%.
This article is a reliable source of information on the comparison between Gradient Boosting Decision Trees (GBDT), Artificial Neural Networks (ANN) and Random Forests (RF) in modeling Land Use Change (LUC) in the Seoul Metropolitan Area. The authors provide a detailed description of their methodology and results, as well as a discussion of their findings. The article is well-written and easy to understand, making it accessible to readers with varying levels of expertise in this field.
The article does not appear to be biased or one-sided; it presents both sides equally and objectively evaluates each method's performance without favoring any particular approach. Furthermore, all claims made are supported by evidence from the study's results, making them trustworthy and reliable.
The only potential issue with this article is that it does not explore any counterarguments or alternative explanations for its findings; however, this is understandable given the scope of the study and its focus on comparing three specific methods rather than exploring broader topics related to LUC modeling.
In conclusion, this article is a reliable source of information on LUC modeling using GBDTs, ANNs and RFs in the Seoul Metropolitan Area; it is well-written, objective, evidence-based and free from promotional content or partiality.