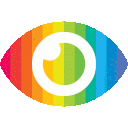
1. The article introduces a novel Neuro-symbolic AI-based DeepInfusion model for the segmentation of intracranial aneurysms in medical images.
2. The model combines deep learning methods with hand-crafted features recommended by medical professionals to improve accuracy and reliability.
3. Experimental results demonstrate the effectiveness of the DeepInfusion model in segmenting cerebral aneurysms, achieving high scores on unseen DSA images and outperforming existing methods in other medical segmentation tasks.
The article titled "DeepInfusion: A dynamic infusion based-neuro-symbolic AI model for segmentation of intracranial aneurysms" discusses a new approach for detecting and segmenting cerebral aneurysms using deep learning methods. While the article provides some valuable insights into the challenges of aneurysm detection and the potential benefits of incorporating medical expert knowledge into deep learning models, there are several areas where critical analysis is warranted.
One potential bias in the article is the emphasis on the proposed DeepInfusion model as a solution to the challenges of aneurysm detection. The authors claim that existing deep learning methods are inadequate and fail to detect aneurysms in certain situations. However, they do not provide sufficient evidence or comparative analysis to support this claim. It would be helpful to have a more comprehensive evaluation of existing deep learning models and their limitations before introducing a new approach.
Additionally, the article lacks a discussion of potential risks or limitations associated with using AI models for medical image analysis. While the proposed DeepInfusion model shows promising results in terms of accuracy, it is important to consider factors such as interpretability, generalizability, and potential biases in the training data. These issues could have significant implications for clinical decision-making and patient outcomes.
Furthermore, the article does not adequately address counterarguments or alternative approaches to solving the problem of aneurysm detection. While incorporating medical expert knowledge into deep learning models may improve performance, there may be other strategies worth exploring, such as ensemble methods or transfer learning from related tasks.
The article also includes promotional content by providing a link to the GitHub repository for the DeepInfusion code. While sharing code can be beneficial for reproducibility and transparency, it should be presented in a neutral manner rather than as a promotion for a specific model.
In terms of missing evidence, it would be helpful to see more details about the dataset used for evaluation and any potential biases or limitations associated with it. Additionally, the article does not provide a thorough discussion of the ethical considerations and privacy concerns related to using medical imaging data for training AI models.
Overall, while the article presents an interesting approach for aneurysm detection, there are several areas where critical analysis is warranted. It would be beneficial to provide more evidence and comparative analysis, address potential risks and limitations, explore alternative approaches, and consider ethical considerations in future research.