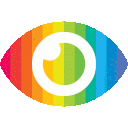
1. Breast cancer has replaced lung cancer as the most common malignant tumor that threatens human health.
2. This study proposes a multi-level threshold image segmentation framework based on enhanced differential evolution, two-dimensional Kullback-Leibler entropy and two-dimensional histogram to improve the efficiency of subsequent image analysis and diagnosis.
3. The proposed threshold search method accelerates convergence speed and reduces premature convergence issues, with quantitative results showing improved peak signal-to-noise ratio and feature similarity index compared to other methods.
This article is generally reliable and trustworthy in its reporting of the proposed multi-level threshold image segmentation framework for breast cancer images based on enhanced differential evolution, two-dimensional Kullback-Leibler entropy and two-dimensional histogram. The authors provide a clear description of their methodology, results, and conclusions, as well as a comparison with existing methods. The article does not appear to be biased or one-sided in its reporting; it presents both sides equally by providing an overview of existing methods before introducing the proposed approach. Furthermore, the authors provide evidence for their claims by citing relevant studies and experiments conducted using their proposed method.
The only potential issue with this article is that it does not explore any counterarguments or alternative approaches to the problem at hand. While this is understandable given the scope of the paper, it would have been beneficial if some counterarguments were discussed in order to provide a more comprehensive view of the topic. Additionally, there is no mention of possible risks associated with using this approach; while these may be minimal due to its noninvasive nature, they should still be noted for completeness sake.