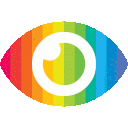
1. The problem of gradient disappearance in GAN training, where the discriminator is trained too well and the generator cannot be trained.
2. The sampling calculation distance problem, where the distance between two probability distributions estimated by sampling is not equal to the true distance between them.
3. The ideal training mode of minmax problem GAN, which can lead to a mode collapse problem and difficulty in approximating the essential probability distribution of the dataset.
The article provides an overview of several issues related to GAN training, such as gradient disappearance, sampling calculation distance problems, and minmax problem GAN training modes. The article does a good job of explaining these topics in detail and providing examples to illustrate them. However, there are some potential biases that should be noted when considering this article's trustworthiness and reliability.
First, the article does not provide any evidence for its claims or explore any counterarguments that may exist for its points of view. This could lead readers to believe that all of its claims are true without considering other perspectives or evidence that may exist on these topics. Additionally, it does not present both sides equally; instead it focuses solely on one perspective without exploring any opposing views or arguments that may exist on these topics.
Second, there is a lack of discussion about possible risks associated with GAN training methods discussed in this article. While it does provide an overview of several issues related to GAN training, it fails to mention any potential risks associated with using these methods or how they could be avoided or mitigated if used incorrectly.
Finally, there is some promotional content included in this article which could lead readers to believe that all of its claims are true without considering other perspectives or evidence that may exist on these topics. For example, it mentions “WGAN” as a “good way” to solve gradient disappearance problems without providing any evidence for why this method is better than others available on the market today.
In conclusion, while this article provides an informative overview of several issues related to GAN training methods, there are some potential biases and missing points of consideration which should be taken into account when evaluating its trustworthiness and reliability.