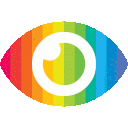
1. The article presents a computational model of hippocampal and dorsal striatal learning and decision making, which explains how humans and animals trade off multiple decision-making strategies.
2. The model distinguishes between stimulus-response (model-free) learning and deliberative (model-based) planning, as well as between response learning and place learning in spatial navigation.
3. The model successfully characterizes the hippocampal-striatal system as a general system for decision making via adaptive combination of stimulus-response learning and the use of a cognitive map.
The article "A general model of hippocampal and dorsal striatal learning and decision making" presents a computational model that unifies findings from the spatial-navigation and decision-making fields. The authors propose that the distinction between place and response learning in spatial navigation is analogous to that between model-based (MB) and model-free (MF) reinforcement learning (RL). They suggest that the hippocampus supports MB learning by representing relational structure in a cognitive map, while the dorsolateral striatum implements MF response learning by learning associations between actions and egocentric representations of landmarks.
The article provides a detailed explanation of the proposed model, including its architecture, components, and computations. The authors show how their model can explain a range of seemingly disparate behavioral findings in spatial and nonspatial decision tasks, as well as the effects of lesions to DLS and hippocampus on these tasks. They also demonstrate how modeling place cells as driven by boundaries explains why navigation guided by boundaries is robust to "blocking" by prior state-reward associations due to learned associations between place cells.
Overall, the article presents a compelling argument for the proposed model's ability to unify findings from different fields of research. However, there are some potential biases and limitations to consider. For example, the authors rely heavily on animal studies to support their claims about the role of hippocampus and DLS in RL. While animal studies can provide valuable insights into neural mechanisms underlying behavior, they may not always generalize to humans or other species.
Additionally, the article does not explore alternative models or counterarguments that could challenge their proposed framework. For instance, some researchers have suggested that MB and MF RL may not be mutually exclusive strategies but rather complementary processes that interact dynamically depending on task demands (51 [source: https://www.pnas.org/doi/10.1073/pnas.2007981117#core-r51]). Similarly, others have argued that both hippocampus and DLS may contribute to both MB and MF RL depending on task context (52 [source: https://www.pnas.org/doi/10.1073/pnas.2007981117#core-r52]).
Furthermore, while the article notes some potential risks associated with relying too heavily on either MB or MF RL strategies (e.g., inflexibility vs. slow learning), it does not discuss potential ethical implications or societal impacts of this research. For example, if this model were applied in artificial intelligence systems or human decision-making contexts, it could have significant consequences for privacy rights, fairness considerations, or social justice issues.
In conclusion, while this article presents an intriguing computational model for understanding RL in spatial navigation and decision-making contexts, it is important to consider its potential biases and limitations carefully. Future research should continue to explore alternative models and counterarguments while also considering broader ethical implications of this work for society at large.