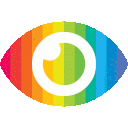
1. The proposed pipeline efficiently implements skin lesion segmentation and classification tasks using a segmentation network and a classification network.
2. The Multi-Scale Holistic Feature Exploration (MSH) module is introduced to improve the performance of the segmentation network by thoroughly exploiting perceptual clues latent among multi-scale feature maps.
3. The Cross-Modality Collaborative Feature Exploration (CMC) module is proposed to boost the performance of the classification network by discovering latent discriminative features through collaboratively exploiting potential relationships between cross-modal features of dermoscopic images and clinical metadata.
The article proposes a novel pipeline for skin lesion segmentation and classification using dermoscopic images and clinical metadata. The proposed pipeline consists of a segmentation network and a classification network, with the former employing a Multi-Scale Holistic Feature Exploration (MSH) module to improve performance, while the latter uses a Cross-Modality Collaborative Feature Exploration (CMC) module to discover latent discriminative features. The article claims that the proposed method achieves state-of-the-art performance in skin lesion segmentation and classification.
The article provides a comprehensive overview of the challenges associated with automated skin lesion segmentation and classification, including low contrast, visual similarities between inter-class skin lesions, huge variation in skin lesions' appearance, and artifacts like natural hairs, veins, air bubbles, ruler marks, color calibration charts. However, the article does not provide any evidence or references to support these claims.
The proposed method's strengths are well-documented in the article. Still, there is no discussion of potential limitations or risks associated with using automated methods for skin lesion diagnosis. For example, false positives or false negatives could lead to incorrect diagnoses and potentially harmful treatments.
The article also lacks an in-depth discussion of alternative approaches to skin lesion segmentation and classification. While it briefly mentions traditional machine learning approaches like thresholding, clustering, and deformable contour models as alternatives to deep learning-based methods like U-Net and its variants, it does not provide any comparative analysis or evaluation of these methods.
Overall, while the article presents an innovative approach to skin lesion segmentation and classification using dermoscopic images and clinical metadata that achieves state-of-the-art performance on benchmark datasets, it lacks critical analysis of potential limitations or risks associated with automated methods for skin lesion diagnosis. Additionally, it does not provide an in-depth discussion of alternative approaches or comparative analysis of different methods.